The growing volume of clinical data in medical imaging slows down identification and analysis of specific features in an image. This reduces the annotation speed at which radiologists and imaging technicians capture, screen, and diagnose patient data.
The demand for artificial intelligence in medical image analysis has drastically grown in the last few years. AI-assisted solutions have emerged to speed up annotation workflows and increase productivity.
NVIDIA announced AI-assisted annotation and transfer learning at Radiological Society of North America (RSNA) 2018, a premier conference that showcases the latest research and technology advances making headlines in radiology. Clara Train SDK contains these tools to speed up AI-assisted workflows for medical imaging.
This post shows how fast annotation works with the transfer learning workflow for medical imaging.
Annotation tools in medical imaging applications help radiologists annotate organs and abnormalities. These applications consist of software tools to draw boundaries around the organs of interest, isolating them from the rest of the dataset. These applications typically operate on 2D slices. A radiologist takes a single 2D image slice from a patient’s scan and marks annotation boundaries and corrections as needed. This manual process repeats for the next slice in which the organ of interest is annotated and corrected again.
Some applications support 3D tools for annotation with region-growing techniques that perform fairly well with certain organs but poorly on other organs. Moreover, both these 2D and 3D tools for annotation do not learn and adapt to the dataset being annotated, which becomes crucial for medical imaging applications as the datasets grow.
Because these workflows are completely manual, the annotation process takes time. A normal computed tomography (CT) or magnetic resonance imaging (MRI) scan of a patient has hundreds of 2D slices to cover a particular organ in 3D. New generation scanners produce increasingly higher resolution scans.
AI-assisted annotation
NVIDIA brings its leadership position in modern artificial intelligence and deep learning to help automate the processing and understanding of images generated by medical scanners. NVIDIA AI-assisted annotation enables deep learning-based applications by providing you with tools that make it possible to speed up the annotation process, helping radiologists save time, and increase productivity (Figure 1).
The Clara Train SDK AI-assisted annotation accelerates the annotation process by enabling application developers to integrate deep learning tools built into the SDK with existing medical imaging viewers , such as MITK, ITK-Snap, 3D Slicer, or a custom-built application viewer. This uses a simple API and requires no prior deep learning knowledge. As a result, radiologists can increase their productivity by analyzing more patient data while still using their existing workflows and familiar tools.
Generating segmentation with deep learning models
With AI-assisted annotation, radiologists don’t have to manually draw on each 2D slice to annotate an organ or an abnormality. Instead, they simply click a few extreme points on a particular organ of interest in a 3D MRI or CT scan and receive auto-annotated results for all the 2D slices of that particular organ.
When a user sends the extreme points to the SDK, a deep learning model receives this as input and returns the inference results of the segmented organ or abnormality. Figure 2 highlights the steps in the process.
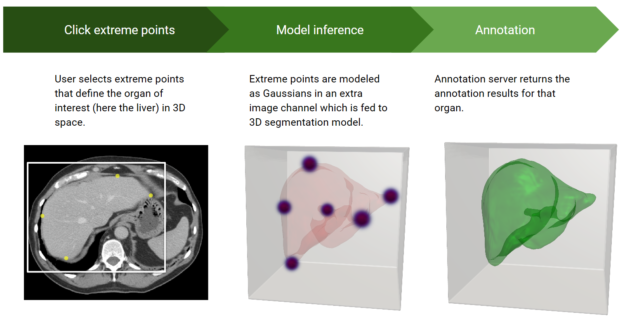
Model registry for organs
The SDK incorporates deep learning models to perform AI-assisted annotation on a particular organ or abnormality. NVIDIA provides 13 different organ models for the early access release, which have all been pretrained on public datasets by NVIDIA data scientists. These models can be used for annotation and can kickstart your development effort by enabling faster annotation of datasets for AI algorithms. Additional organ models are under development.
Polygon editing
Because deep learning models are by their nature sensitive to the data used to train them, annotation accuracy might be lower than originally achieved with the training data.
If there are 2D slices with incorrect or partial annotation, 2D smart polygon editing feature helps in correcting these slices. When you move a single polygon point on the 2D slice, all other points around a radius automatically snap to the organ boundaries, making it easier and efficient when correcting (Figure 3).
The following section describes how to save these corrected slices as new ground-truth and use them to retrain the annotation model, consistently increasing annotation accuracy.
Transfer learning workflow
Deep learning models are sensitive to the data used to train them, as described earlier. Factors such as varying scanner configurations, age differences of patients, and so on, must be taken into account. This makes it hard to train the deep learning models on a specific dataset and deploy them on a different dataset.
Using transfer learning is well-suited for medical image analysis. As medical image analysis is a computer vision task, CNNs represent the best performing methods for this. Getting a large well-annotated dataset is considerably harder in the medical domain, compared to the general computer vision domain, because of the domain expertise required to annotate the medical images. This makes transfer learning a natural fit for medical image analysis. Use a pretrained CNN on a larger database and then apply transfer learning to a target domain of medical images with limited availability.
As a solution, transfer learning for medical imaging helps you adapt pretrained models into your datasets. Deep learning models used for annotation can be tuned and improved by retraining these pretrained models based on new datasets (Figure 4).
Clara Train SDK contains Annotation SDK and Transfer Learning Toolkit and is available in a Docker container.
NVIDIA Transfer Learning Toolkit (TLT) provides domain-specific pretrained models for intelligent video analytics. The same underlying technology allows users to adapt medical imaging segmentation DNNs. Clara Train SDK for Medical Imaging contains several models from the public domain, optimized in-house to achieve high accuracy. Medical imaging developers can choose from one of the provided models as a starting point and use a high-level Python SDK to retrain and adapt these models with custom data.
We provide Python wrappers for model retraining and exporting. These models are trained using TensorFlow framework on public datasets. The complexity of adaptation is considerably reduced because of easy-to-use shell commands, data conversion guidance, and an export wrapper for converting trained models to a TensorRT-optimized graph.
A deep learning image segmentation approach is used for fine-grained predictions needed in medical imaging. Image segmentation algorithms partition an input image into multiple segments. Images become divided down to the voxel level (a volumetric pixel is the 3-D equivalent of a pixel) and each pixel gets assigned a label or is classified. For every pixel in the image, the network is trained to predict the pixel class. This enables the network to both identify several object classes in each image and determine the location of objects. Image segmentation generates a label image the same size as the input, in which the pixels are color-coded according to their classes.
Accurate segmentation is key in various medical applications.
Using pretrained models
The medical segmentation decathlon challenge site provides a reliable dataset starting point for segmentation model development. All segmentation models in the SDK are trained from the identical segmentation training pipeline, with configurations for brain, heart, pancreas, and spleen segmentation. Because several models have been trained with images 1x1x1mm resolution, data needs to be converted to 1x1x1mm NIfTI format. The site provides easy-to-use conversion utilities.
Models can be integrated into other applications. The toolkit supports applications built using the NVIDIA Clara developer platform and deployed for inference with the TensorRT Inference Server. To learn more about how to integrate AI-assisted annotation into your existing application, see the NVIDIA Clara documentation.
Annotate faster with Clara Train
Get started using Clara Train SDK today. Easily develop, train, and adapt your deep learning models. You can begin with the AI Assisted Annotation Client and Reference plugin by downloading the client software. The Docker container that includes Python SDK and Annotation Server can be downloaded from NGC. Model applications can also be downloaded from NGC.