The hottest area in machine learning today is Deep Learning, which uses Deep Neural Networks (DNNs) to teach computers to detect recognizable concepts in data. Researchers and industry practitioners are using DNNs in image and video classification, computer vision, speech recognition, natural language processing, and audio recognition, among other applications.
The success of DNNs has been greatly accelerated by using GPUs, which have become the platform of choice for training these large, complex DNNs, reducing training time from months to only a few days. The major deep learning software frameworks have incorporated GPU acceleration, including Caffe, Torch7, Theano, and CUDA-Convnet2. Because of the increasing importance of DNNs in both industry and academia and the key role of GPUs, last year NVIDIA introduced cuDNN, a library of primitives for deep neural networks.
Today at the GPU Technology Conference, NVIDIA CEO and co-founder Jen-Hsun Huang introduced DIGITS, the first interactive Deep Learning GPU Training System. DIGITS is a new system for developing, training and visualizing deep neural networks. It puts the power of deep learning into an intuitive browser-based interface, so that data scientists and researchers can quickly design the best DNN for their data using real-time network behavior visualization. DIGITS is open-source software, available on GitHub, so developers can extend or customize it or contribute to the project.
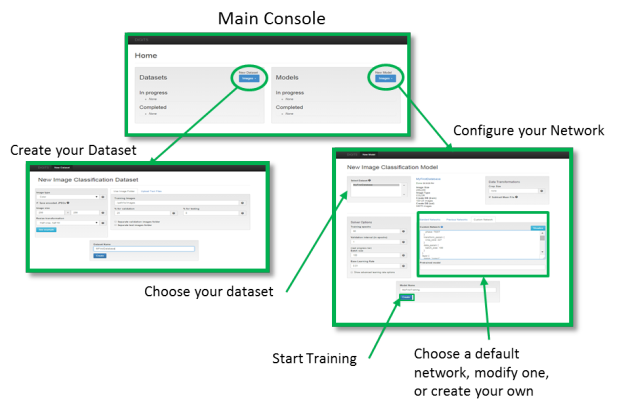
Deep Learning is an approach to training and employing multi-layered artificial neural networks to assist in or complete a task without human intervention. DNNs for image classification typically use a combination of convolutional neural network (CNN) layers and fully connected layers made up of artificial neurons tiled so that they respond to overlapping regions of the visual field.
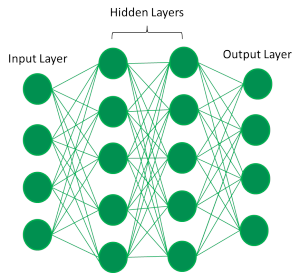
Figure 2 shows a generic representation of a network with two hidden layers showing the interactions between layers. Feature processing occurs in each layer, represented as a series of neurons. Links between the layers communicate responses, akin to synapses. The general approach of processing data through multiple layers, performing feature abstraction at each layer, is analogous to how the brain processes information. The number of layers and their parameters can vary depending on the data and categories. Some deep neural networks are comprised of more than ten layers with more than a billion parameters [1][2].
Advantages of DIGITS
DIGITS provides a user-friendly interface for training and classification that can be used to train DNNs with a few clicks. It runs as a web application accessed through a web browser. Figure 1 shows the typical user work flow in DIGITS. The first screen is the main console window, from which you can create databases from images and prepare them for training. Once you have a database, you can configure your network model and begin training.
The DIGITS interface provides tools for DNN optimization. The main console lists existing databases and previously trained network models available on the machine, as well as the training activities in progress. You can track adjustments you have made to network configuration and maximize accuracy by varying parameters such as bias, neural activation functions, pooling windows, and layers.
DIGITS makes it easy to visualize networks and quickly compare their accuracies. When you select a model, DIGITS shows the status of the training exercise and its accuracy, and provides the option to load and classify images while the network is training or after training completes.
Because DIGITS runs a web server, it is easy for a team of users to share datasets and network configurations, and to test and share results. Within an organization several people may use the same data set for training networks with different configurations.
DIGITS integrates the popular Caffe deep learning framework from the Berkeley Learning and Vision Center, and supports GPU acceleration using cuDNN to massively reduce training time.
Installing DIGITS
Installing and using DIGITS is easy. Visit the digits home page, register and download the installer. Or, if you prefer, get the (Python-based) source code from Github.
Using DIGITS
Once everything is installed, launch DIGITS from its install directory using this command line:
python digits-devserver
Then, if DIGITS is installed on your local machine, load the DIGITS web interface in your web browser by entering the URL http://localhost:5000. If it is installed on a server you can replace localhost
with the server IP address or hostname.
When you first open the DIGITS main console it will not have any databases, as shown in Figure 2. Creating a database is easy. Select “Images” under “New Dataset” in the left pane. You have two options for creating a database from images: either add the path to the “Training Image” text box and let DIGITS create the training and validation sets, or insert paths to both sets using the “Upload Text Files” tab. Once the database paths have been defined use the “Create” button to generate the database.
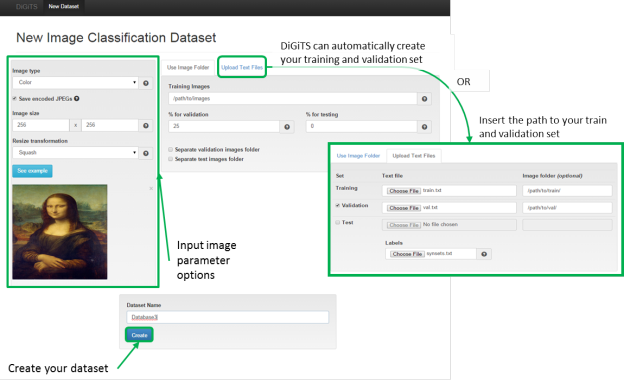
After creating a database you should define the network parameters for training. Go back to the main console and select any previously created dataset under “New Model”. In Figure 4 we have selected “Database1” from the two available datasets. Many of the features and functions available in the Caffe framework are exposed in the “Solver Options” pane on the left side. All network functions are available as well.
You have three options for defining a network: selecting a preconfigured (“standard”) network, a previous network, or a custom network, as shown in Figure 4 (middle). LeNet by Yann LeCunn and AlexNet from Alex Krizhevsky are the two preconfigured networks currently available. You can also modify these networks by selecting the customize link next to the network. This lets you modify any of the network parameters, add layers, change the bias, or modify the pooling windows.
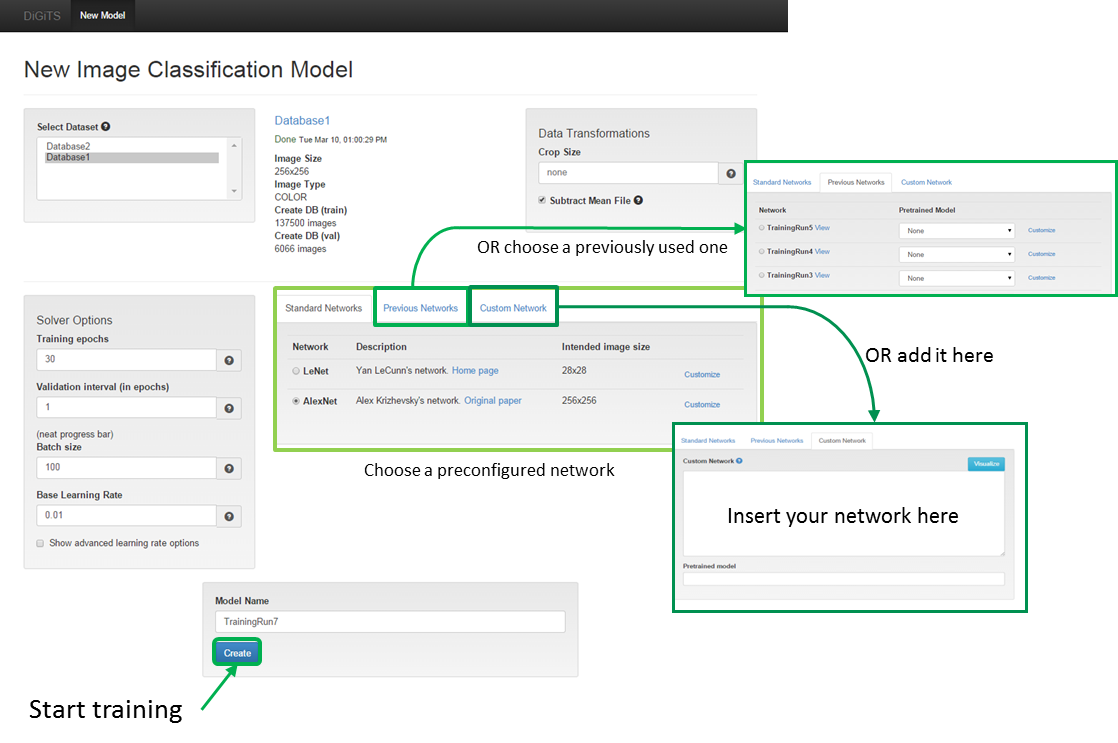
When you customize the network you can visualize it by selecting the “Visualize” button in the upper-right corner of the network editing box. This is a handy network configuration checking tool that helps you visualize your network layout and quickly tells you if you have the wrong inputs into certain layers or forgot to put in a pooling function.
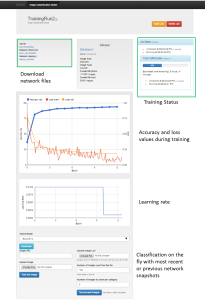
After the configuration is complete you can start training! Figure 5 shows training results for a two-class image set using the the example caffenet network configuration. The top of the training window has links to the network configuration files, information on the data set used, and training status. If an error occurs during training it is posted in this area. You can download the network configuration files from this window to quickly check parameters during training.
During training DIGITS plots the accuracy and loss values in the top chart. This is handy because it provides real-time visualization into how well or poorly the network is learning. If the accuracy is not increasing or is not as expected you can abort training and/or delete it using the buttons in the upper-right corner. The learning rate as a function of the training epoch is plotted in the lower plot.
You can classify images with the network using the interface below the plots. Like Caffe, DIGITS takes snapshots during training; you can use the most recent (or any previous) snapshot to classify images. You can select a snapshot with the “Select Model” drop-down menu and choosing your desired Epoch. Then simply input the URL of an online image or upload one from your local computer, and click “Test One Image”. You can also classify multiple images at once by uploading a text file with a list of URLs or images located on the host machine.
How I use DIGITS
Let’s demonstrate DIGITS with a look at a test network and how I used it to put it through its paces. I chose a relatively simple task: identifying images of ships. I started with two categories, “ship” and “no ship”. I obtained approximately 34,000 images from ImageNet via the URL lists provided on the site and by manually searching on USGS. ImageNet images are pre-tagged, which made it easy to categorize the images I found there. I manually tiled and tagged all of the USGS images. My ship category comprises a variety of different marine vehicles including cruise, cargo, weather, passenger and container ships; oil tankers, destroyers, and small boats. My non-ship category includes images of beaches, open water, buildings, sharks, whales, and other non-ship objects.
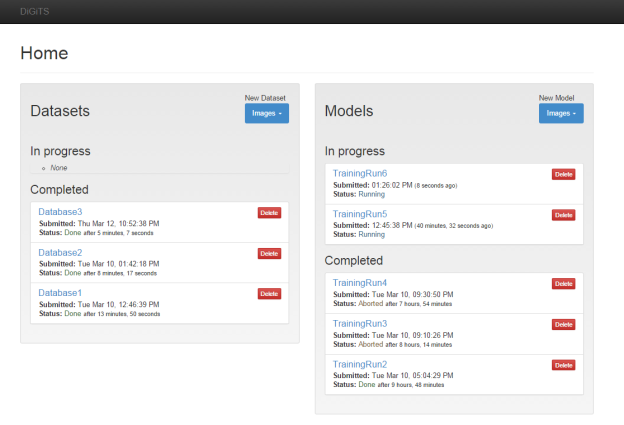
I was a Caffe user, and DIGITS was immediately useful thanks to the user-friendly interface and the access it provides to Caffe features.
I have multiple datasets built from the same image data. One set comprises my original images and the others are modified versions. In the modified versions I mirrored all of the training images to inflate the dataset and try to account for variation in object orientations. I like that DIGITS displays all of my previously created datasets in the Main Console, and also in the network window, making it easy to select the one I want to use in each training exercise. It’s also easy to modify my networks, either by downloading a network file from a previous training run and pasting the modified version into the custom network box, or by loading one of my previous networks and customizing it.
DIGITS is great for sharing data and results. I live in LA and work with a team in Texas and the Washington DC area. We run DIGITS on an internal server that everyone can access. This allows us to quickly check and track the iterations on our network configurations and see how changes affect network performance. Anyone with access can also configure their own network in DIGITS and perform CNN training on this host. Their activities display in the main console too. To demonstrate, Figure 6 shows an image of my current console. Three datasets I currently have stored as well as complete and current models are posted.
DIGITS makes it easy for me to visualize my network when I classify an image. When classifying a single image it displays the activations at each layer as well as the kernels. I find it hard to mentally visualize a network’s response, but this feature helps by concisely showing all of the layer and activation information. Figure 7 shows an example of my test network correctly classifying an old photo of a military ship with 100% confidence, and Figure 8 shows the results of classifying a picture of me. It shows that my two-class ship/no-ship neural network is 100% sure that I am not a ship!
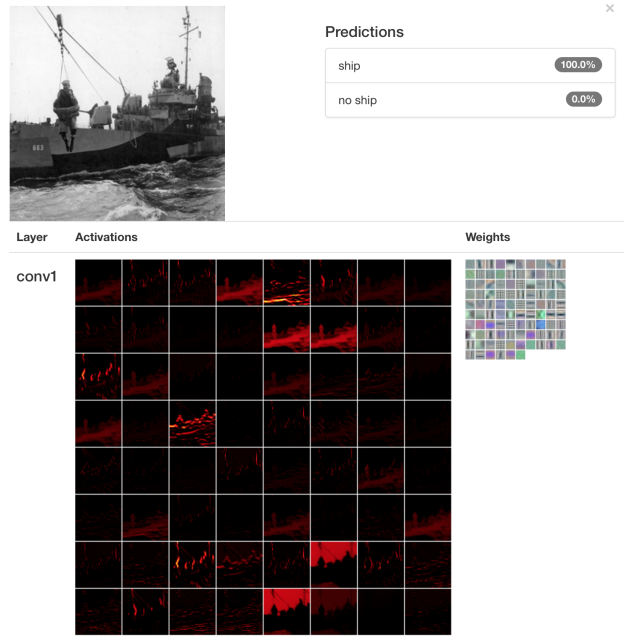
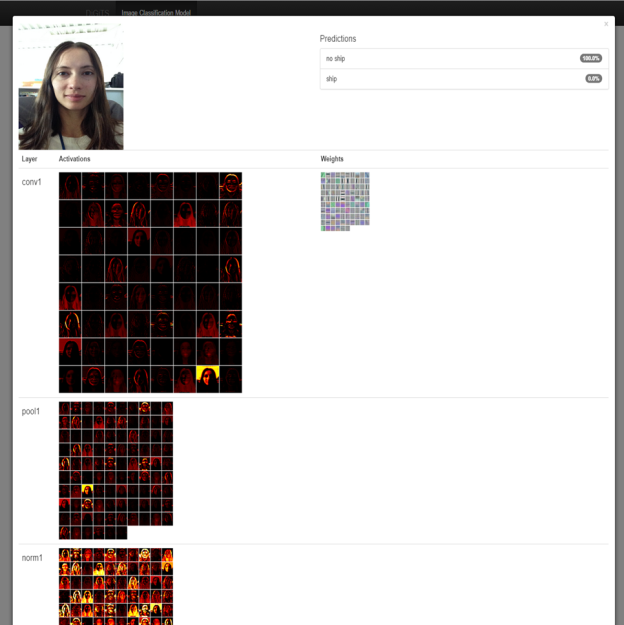
References
[1] Krizhevsky, A., Sutskever, I. and Hinton, G. E., ImageNet Classification with Deep Convolutional Neural Networks. NIPS 2012: Neural Information Processing Systems, Lake Tahoe, Nevada.
[2] Szegedy, C. et al., Going Deeper with Convolutions. September 12, 2014. http://arxiv.org/pdf/1409.4842v1.pdf